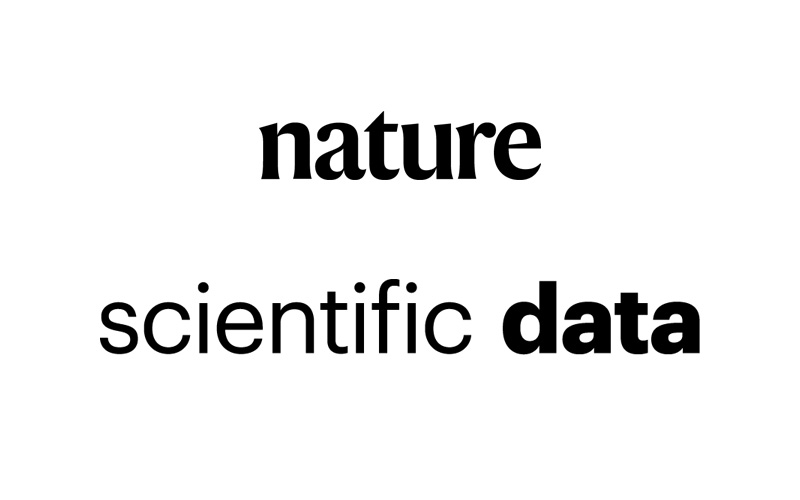
The Building Data Genome Project 2:
Energy meter data from the ASHRAE Great Energy Predictor III competition
NATURE, Scientific Data 7, article number: 368 (2020), October 2020
Authors: Clayton Miller a ; Anjukan Kathirgamanathan b ; Bianca Picchetti c et al.
a Building and Urban Data Science (BUDS) Lab, School of Design and Environment (SDE), National University of Singapore (NUS), 4 Architecture Drive, Singapore 117566, Singapore
b UCD Energy Institute, O’Brien Science Building, University College Dublin, Belfield, Dublin, D04 V1W8, Ireland
c Gerencia del Ciclo de Combustible Nuclear, Comisión Nacional de Energía Atómica, Avenida General Paz 1499, Buenos Aires, 1650, Argentina
OPEN ACCESS
Abstract:
This paper describes an open data set of 3,053 energy meters from 1,636 non-residential buildings with a range of two full years (2016 and 2017) at an hourly frequency (17,544 measurements per meter resulting in approximately 53.6 million measurements). These meters were collected from 19 sites across North America and Europe, with one or more meters per building measuring whole building electrical, heating and cooling water, steam, and solar energy as well as water and irrigation meters.
Part of these data was used in the Great Energy Predictor III (GEPIII) competition hosted by the American Society of Heating, Refrigeration, and Air-Conditioning Engineers (ASHRAE) in October-December 2019. GEPIII was a machine learning competition for long-term prediction with an application to measurement and verification.
This paper describes the process of data collection, cleaning, and convergence of time-series meter data, the meta-data about the buildings, and complementary weather data. This data set can be used for further prediction benchmarking and prototyping as well as anomaly detection, energy analysis, and building type classification.
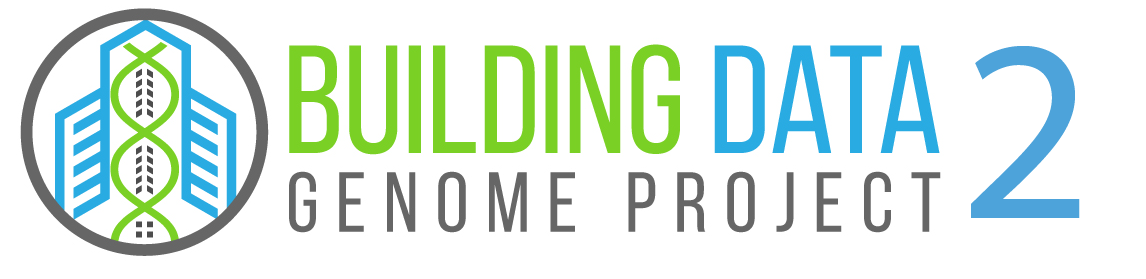
The Building Data Genome Project 2 (BDG2) is an open data set made up of 3,053 energy meters from 1,636 buildings. The time range of the times-series data is the two full years (2016 and 2017) and the frequency is hourly measurements of electricity, heating and cooling water, steam, and irrigation meters.
The data set can be used to benchmark various statistical learning algorithms and other data science techniques. It can also be used simply as a teaching or learning tool to practice dealing with measured performance data from large numbers of non-residential buildings.
The source code and data sets are available at GitHub and includes instructions and guide to use it.